– Olga, your field of activity is bioinformatics, you create neural networks for geneticists and biologists. What exactly can artificial intelligence be used for in this field?
— Today, there are many areas for the application of artificial intelligence in biology. For example, from a photo of a child with its help, it can be assumed how rare a genetic disease he has. There is such an application from the company Face2Gene: we take a photo, upload it, and the neural network analyzes the child’s face. It gives geneticists clues as to which genes to look for mutations in to accurately diagnose a child.
Neural networks began to emerge that told bioinformatics translators how much pathology a mutation that didn’t code for our proteins could have.
What is a “non-coding protein” mutation?
– Our genome consists of two parts: segments that code for a protein (responsible for its production in the body) and segments of non-coding, so-called “junk” DNA. Contrary to its name, it is not useless – its function includes, among other things, the regulation of genes.
The mutation we are talking about is in the non-coding region of the genome. Such mutations are difficult to interpret: we cannot say with certainty whether these changes in the genome will affect the development of the disease. In general, it is worth noting here that not every change in the genome is pathogenic. Therefore, the dreaded word “mutation” that signals us to something bad is replaced by the word “variant” in the professional community. And variants can already be interpreted as benign, pathogenic and of unknown clinical significance. The vast majority of variants in our genetic code do not cause any disease.
There are already clear guidelines on how to determine the pathogenicity of variants in the region that encodes proteins.
— And how can a neural network help with that?
— We created a DeepCT neural network that suggests the role of mutation in different cell types.
We have the same DNA sequence in every cell in our body. But at the same time, from some cells we develop a kidney, from others – a heart, from a third – a brain. Why does this happen in the same order? Because we have some kind of gene editing program. Some genes are turned off and do not work, while others are turned on. For example, the genes responsible for the active functioning of the heart muscle do not work in brain cells.
DeepCT is not yet a 100% clinical tool, but it can predict the role of mutations. The results of the neural network predictions still need to be verified.
Google also has an Informer tool based on neural networks; this allows you to predict the expression (activity) of a particular gene in general, not just the association of a gene with some proteins. Informer answers the question: Does a mutation in a particular gene affect protein synthesis? Will the gene be expressed in cells?
— Do not Russian scientists have such developments?
– To eat. Inspired by him, we decided to create our own analogue, Deep CT. There are now language models for DNA similar to natural language processing models. But the truth is that existing neural networks were either in private access or didn’t work very well. We have trained our model, which we call GENA, it is available to all scientists around the world. The program can take very small DNA sequences and learn the patterns in them.
Can it analyze long segments? If we are talking about DNA analysis, what generally affects the length of the segment?
– The length of the array is very important because you need to understand the context where the mutations happen. The longer we give the sequence, the more the neural network can see and learn some patterns.
Our task is to increase the sequence that the neural network can analyze. The first version of GENA runs about 3000 nucleotides long, while the second architecture of the model allows 24000 nucleotides to be fed into the input. Both models are already public and available to the world community. Over the past month, researchers around the world have downloaded the two solutions more than 1,000 times.
We have our own new proprietary architecture called RMT – Repetitive Memory Transformer, which was developed at AIRI together with our colleagues from the Moscow Institute of Physics and Technology. It can work with text strings of potentially unlimited length; successfully copes with lengths of 1-2 million letters in a series of tasks. In experiments on genomic data, we are currently investigating the ability to process more than 24,000 letters. Now we are preparing to write a scientific article and present it officially.
— Has GENA already identified previously unknown patterns associated with mutations?
— Yes, GENA pays attention to areas of biological significance. So even if we can’t find any statement in the scientific literature that a particular region of the genome affects something, that doesn’t mean the neural network is faulty. Maybe he found something new unknown in biology.
Such areas need to be studied – they will help slowly unravel the mysteries of our genome.
We are also finding new approaches to the use of GENA. For example, we want to apply this to metagenomic community sequencing data from bat droppings. We’re testing to see if GENA can identify the different virus and bacterial genomes sequenced in this litter. But all of them are still in the idea stage.
– Are there neural networks that can predict the occurrence of genetic diseases in an unborn person?
— These neural networks are currently under development.
Generally, it is recommended that any couple planning to have children go to a geneticist. It is the geneticist who can suggest whether there is a risk of carrying some rare monogenic (when the disease is caused by a mutation in a gene) disease. This is done without neural networks.
If a pathogenic mutation in a gene associated with a known disease is found in healthy parents, the risk of having a sick child is 25%. Such parents are recommended to undergo an IVF procedure with preimplantation genetic screening for this mutation. However, it is also possible to check the genetic profile of the embryo for all chromosomes.
This screening is a genetic test in which embryos are examined before they are transferred to the uterine cavity. Several cells are taken from these embryos, and the programs identify deviations in the genetic profile of the future fetus. Today these programs are based on well-known mathematical algorithms. Today, neural networks are included in them for a more complete analysis of the entire genomic profile of the embryo, but now all this is at an early stage.
Are you talking about the situation in the world or in Russia?
— Let me tell you a little secret: In Russia, there is a team at the Institute of Cytology and Genetics of the Siberian Branch of the Russian Academy of Sciences that has created its own method for embryo screening. It is different from those on the market. While this study is in scientific development, testing is ongoing on a variety of synthetic and non-embryonic living specimens containing some types of aneuploidies (numerical chromosomal abnormalities).
How exactly does this method differ from analogues?
— I can’t explain all the details, but the technology there is completely different. What’s more, it doesn’t just allow DNA sequencing, it just doesn’t allow to read its sequence and determine exactly where the mutation occurred. Colleagues plan to add the ability to see how these chromosomes are positioned in the 3D structure of our nucleus. In these changes, we will be able to see which gene comes into contact with which gene and how different regulatory processes can come into play.
In other words, is this a more complete analysis?
Yes, it has been extended.
— Can neural networks help in the study of coronavirus?
— And yes, this is a very interesting aspect. Neural networks can help predict the next mutation of the coronavirus (so far, current models for this task unfortunately don’t work very well) and suggest how the property of the vaccine could be improved for the new strain.
As you know, the S-protein (the first protrusion that comes into contact with the cell) is very important in the structure of the coronavirus. This is exactly the protein found in the structure of the coronavirus and to which antibodies are produced. The places where antibodies “sit” are called epitopes.
If the virus mutates and changes affect these epitopes, unfortunately, immunity developed after vaccination or after infection with a previous strain of coronavirus will be either less effective or even ineffective.
Together with our colleagues from the Gamaleya Center, we created the SEMA neural network, which allows you to load the virus protein sequence as input and get data on where these epitopes are located.
– What if these places have changed in the new version of the virus?
– Then we can unfortunately assume that these epitopes are no longer effective. Therefore, the vaccine should be updated.
We also developed the model. Now lets you determine if there are glycans on the protein surface. These are polysaccharides that coat the protein like “bushes”. It is a natural barrier against antibody binding. That is, antibodies cannot bind to the epitope if there are polysaccharides on top.
In other words, SEMA can be used to evaluate biotechnological developments, how immunogenic a protein is, and which antibodies are produced. And to evaluate whether a drug based on some type of antibody against the virus would work.
Does it only work with coronavirus?
“It was designed for him, yes, but now you can study any virus with it.
What developments are you currently working on?
“Now in the world there are neural networks that help create a completely new, biologically active protein that did not exist before in nature. is heard.
We are now creating our own similar model, but so far only in the initial phase of development.
Source: Gazeta
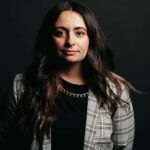
Barbara Dickson is a seasoned writer for “Social Bites”. She keeps readers informed on the latest news and trends, providing in-depth coverage and analysis on a variety of topics.